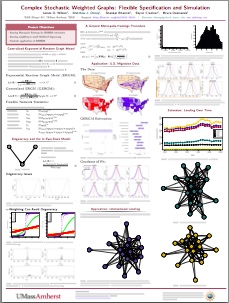
Complex Stochastic Weighted Graphs: Flexible Specification and Simulation
Presented at the International Conference on Computational Social Science , Helsinki Finland, 2015.
For more than half a century, scholars have been studying legislative effectiveness using a single metric -- whether the bills a member sponsors progress through the legislative process. We investigate a less orthodox form of effectiveness -- bill proposals that become law as provisions of other bills. Counting these "hitchhiker" bills as additional cases of bill sponsorship success reveals a more productive, less hierarchical and less partisan lawmaking process. We argue that agenda and procedural constraints are central to understanding why lawmakers pursue hitchhiker strategies. We also investigate the legislative vehicles that attract hitchhikers and find, among other things, that more Senate bills are enacted as hitchhikers on House laws than become law on their own.
Despite the popularity of unsupervised techniques for political science text-as-data research, the importance and implications of preprocessing decisions in this domain have received scant systematic attention. Yet, as we show, such decisions have profound effects on the results of real models for real data. We argue that substantive theory is typically too vague to be of use for feature selection, and that the supervised literature is not necessarily a helpful source of advice. To aid researchers working in unsupervised settings, we introduce a statistical procedure and software that examines the sensitivity of findings under alternate preprocessing regimes. This approach complements a researcher’s substantive understanding of a problem by providing a characterization of the variability changes in preprocessing choices may induce when analyzing a particular dataset. In making scholars aware of the degree to which their results are likely to be sensitive to their preprocessing decisions, it aids replication efforts.
We investigate the functional organization of the Default Mode Network (DMN) – an important subnetwork within the brain associated with a wide range of higher-order cognitive functions. While past work has shown the whole-brain network of functional connectivity follows small-world organizational principles, subnetwork structure is less well understood. Current statistical tools, however, are not suited to quantifying the operating characteristics of functional networks as they often require threshold censoring of information and do not allow for inferential testing of the role that local processes play in determining network structure. Here, we develop the correlation Generalized Exponential Random Graph Model (cGERGM) – a statistical network model that uses local processes to capture the emergent structural properties of correlation networks without loss of information. Examining the DMN with the cGERGM, we show that, rather than demonstrating small- world properties, the DMN appears to be organized according to principles of a segregated highway – suggesting it is optimized for function-specific coordination between brain regions as opposed to information integration across the DMN. We further validate our findings through assessing the power and accuracy of the cGERGM on a testbed of simulated networks representing various commonly observed brain architectures.
In most domains of network analysis researchers consider networks that arise in nature with weighted edges. Such networks are routinely dichotomized in the interest of using available methods for statistical inference with networks. The generalized exponential random graph model (GERGM) is a recently proposed method used to simulate and model the edges of a weighted graph. The GERGM specifies a joint distribution for an exponential family of graphs with continuous-valued edge weights. However, current estimation algorithms for the GERGM only allow inference on a restricted family of model specifications. To address this issue, we develop a Metropolis–Hastings method that can be used to estimate any GERGM specification, thereby significantly extending the family of weighted graphs that can be modeled with the GERGM. We show that new flexible model specifications are capable of avoiding likelihood degeneracy and efficiently capturing network structure in applications where such models were not previously available. We demonstrate the utility of this new class of GERGMs through application to two real network data sets, and we further assess the effectiveness of our proposed methodology by simulating non-degenerate model specifications from the well-studied two-stars model. A working R version of the GERGM code is available in the supplement and is incorporated in the GERGM CRAN package.
Sunshine laws establishing government transparency are ubiquitous in the United States; however, the intended degree of openness is often unclear or unrealized. Although researchers have identified characteristics of government organizations or officials that affect the fulfillment of public records requests, they have not considered the influence that government organizations have on each other. This picture of independently acting organizations does not accord with the literature on diffusion in public policy and administration. In this article, we present a field experiment to test whether a county government's fulfillment of a public records request is influenced by the knowledge that its peers have already complied. We argue that knowledge of peer compliance should (1) induce competitive pressures to comply and (2) resolve legal ambiguity in favor of compliance. We find evidence of peer conformity effects both in the time to initial response and in the rate of complete request fulfillment.
Social scientists who do not have specialized natural language processing training often use a unigram bag-of-words (BOW) representation when analyzing text corpora. We offer a new phrase-based method, NPFST, for enriching a unigram BOW. NPFST uses a part-of-speech tagger and a finite state transducer to extract multiword phrases to be added to a unigram BOW. We compare NPFST to both n-gram and parsing methods in terms of yield, recall, and efficiency. We then demonstrate how to use NPFST for exploratory analyses; it performs well, without configuration, on many different kinds of English text. Finally, we present a case study using NPFST to analyze a new corpus of U.S. congressional bills.
Understanding the causal impact of beliefs on contributions in Threshold Public Goods (TPGs) is particularly important since the social optimum can be supported as a Nash Equilibrium and best-response contributions are a function of beliefs. Unfortunately, investigations of the impact of beliefs on behavior are plagued with endogeneity concerns. We create a set of instruments by cleanly and exogenously manipulating beliefs without deception. Tests indicate that the instruments are valid and relevant. Perhaps surprisingly, we fail to find evidence that beliefs are endogenous in either the one-shot or repeated-decision settings. TPG allocations are determined by a base contribution and beliefs in a one shot-setting. In the repeated-decision environment, once we instrument for first-round allocations, we find that second-round allocations are driven equally by beliefs and history. Moreover, we find that failing to instrument prior decisions overstates their importance.
[article], [supporting information], [replication data], [BibTeX]
Bureaucratic agencies have discretion when implementing laws. Because legislators and bureaucratic officials may have different policy preferences, legislators have developed a number of methods to control those officials. Previous research suggests that legislators who are ideologically aligned with the executive branch are more likely to grant the bureaucracy discretion in policy implementation because of their shared policy goals. This is referred to as the "Ally Principle". However, sometimes ideologically aligned legislators place significant constraints on bureaucratic agencies. Why do these legislators curb discretion from supposedly allied agencies? I argue that statutory constraints are a policy tool, and that Republican legislators use them in an effort to reduce the scope of government bureaucracy. I then test this theory using the text from twenty-four years of U.S. Congressional legislation. I find that while Democrats' behavior is consistent with the Ally Principle, Republican legislation displays strong evidence of a "reverse" Ally Principle.
Network representations, theories, and methods have emerged as a powerful framework for examining political phenomena at a systems level. This article illustrates the importance of specifying and testing theories about the structure of political networks at multiple levels, and the potential pitfalls of failing to model the processes by which these networks were generated. These pitfalls range from missing important alternative explanations for an observed network structure, to specifying a model which does not test the hypotheses that derive from the researcher’s theory. It then combines these insights into a general framework for assessing the structural properties of political networks. The framework is applied to re-specify an existing political science study that seeks to engage with network concepts. I find that the approach taken in this paper can dramatically affect inferences about the structure of a political system.
Interpersonal influence plays an important role in determining what legislation will see action the United States Congress. Building on theories that conceptualize a legislator’s influence as an individual property, I cast influence in a relational framework, recognizing that influence is exercised through legislators’ social and political networks. I develop a novel measure of legislative influence using temporal patterns in bill cosponsorship data as an instrument to infer a latent network of influence relationships between legislators. I then validate the measure of legislative influence I derive from these networks in an extension of a recently published study and in the context of predicting bill advancement. I find that my measure performs like an effective measure of interpersonal influence in Congress.
This article introduces a concept and measure of graph compartmentalization. This new measure allows for principled comparison between graphs of arbitrary structure, unlike existing measures such as graph modularity. The proposed measure is invariant to graph size and number of groups and can be calculated analytically, facilitating measurement on very large graphs. I also introduce a block model generative process for compartmentalized graphs as a benchmark on which to validate the proposed measure. Simulation results demonstrate improved performance of the new measure over modularity in recovering the degree of compartmentalization of graphs simulated from the generative model. I also explore an application to the measurement of political polarization.
Presented at the International Conference on Computational Social Science , Helsinki Finland, 2015.
Presented at PolMeth XXXI , University of Georgia, 2014.
Presented at UMass Amherst Computational Social Science Initiative Poster Session, 2014
Sunbelt XXXII, annual conference of the International Network for Social Network Analysis (INSNA), Redondo Beach CA, 2012.
Presented at the NYU NLP and Text as Data Speaker Series, February 16th, 2017.
Presented at New Directions in Analyzing Text as Data , Northeastern University, 2016.
Presented at the 9th Annual Political Networks Conference , Washington University St. Louis, 2016.
Presented at the 9th Annual Political Networks Conference , Washington University St. Louis, 2016.
Presented at the International Conference on Computational Social Science , Helsinki Finland, 2015.
Presented at the International Conference on Computational Social Science , Helsinki Finland, 2015.